Profile
![]() |
Dr. Lin Gao |
Publications
PRS-Net: Planar Reflective Symmetry Detection Net for 3D Models
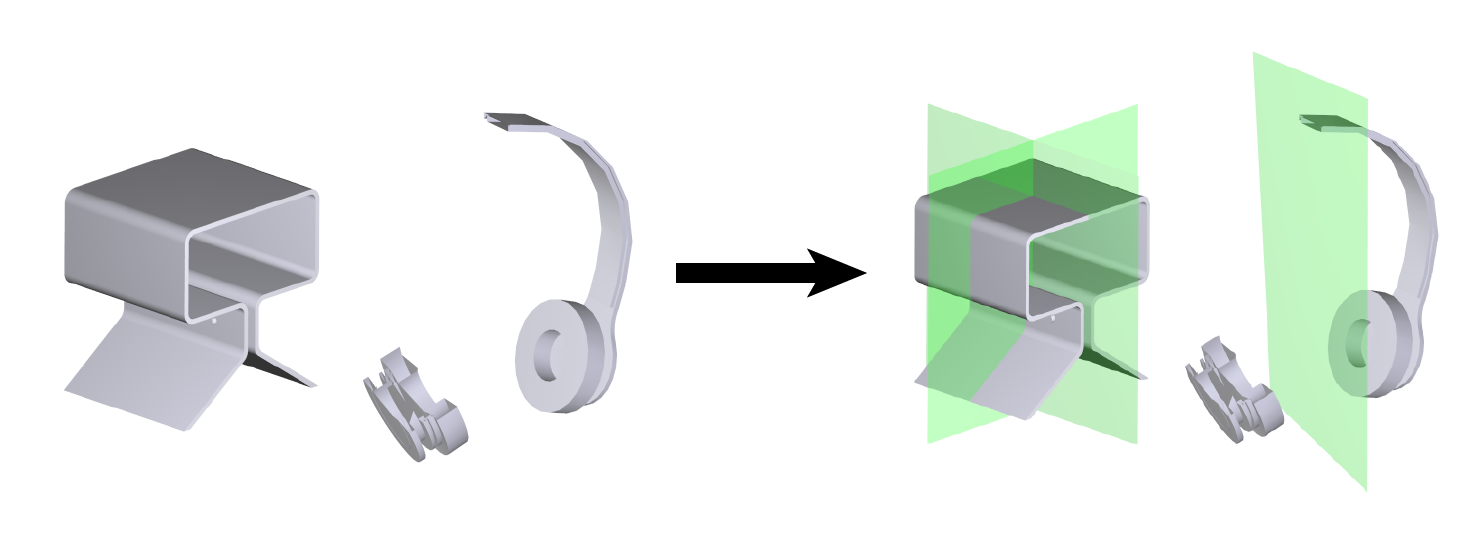
In geometry processing, symmetry is a universal type of high-level structural information of 3D models and benefits many geometry processing tasks including shape segmentation, alignment, matching, and completion. Thus it is an important problem to analyze various symmetry forms of 3D shapes. Planar reflective symmetry is the most fundamental one. Traditional methods based on spatial sampling can be time-consuming and may not be able to identify all the symmetry planes. In this paper, we present a novel learning framework to automatically discover global planar reflective symmetry of a 3D shape. Our framework trains an unsupervised 3D convolutional neural network to extract global model features and then outputs possible global symmetry parameters, where input shapes are represented using voxels. We introduce a dedicated symmetry distance loss along with a regularization loss to avoid generating duplicated symmetry planes. Our network can also identify generalized cylinders by predicting their rotation axes. We further provide a method to remove invalid and duplicated planes and axes. We demonstrate that our method is able to produce reliable and accurate results. Our neural network based method is hundreds of times faster than the state-of-the-art methods, which are based on sampling. Our method is also robust even with noisy or incomplete input surfaces.
@article{abs-1910-06511,
author = {Lin Gao and
Ling{-}Xiao Zhang and
Hsien{-}Yu Meng and
Yi{-}Hui Ren and
Yu{-}Kun Lai and
Leif Kobbelt},
title = {PRS-Net: Planar Reflective Symmetry Detection Net for 3D Models},
journal = {CoRR},
volume = {abs/1910.06511},
year = {2019},
url = {http://arxiv.org/abs/1910.06511},
archivePrefix = {arXiv},
eprint = {1910.06511},
}
ACAP: Sparse Data Driven Mesh Deformation
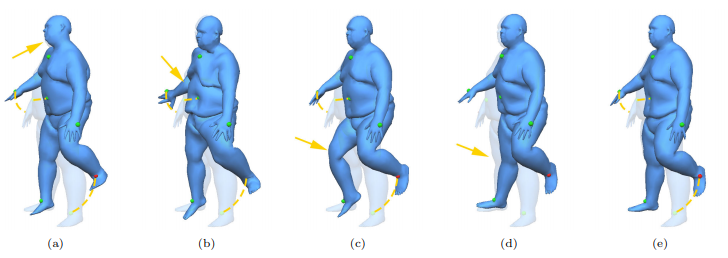
Example-based mesh deformation methods are powerful tools for realistic shape editing. However, existing techniques typically combine all the example deformation modes, which can lead to overfitting, i.e. using an overly complicated model to explain the user-specified deformation. This leads to implausible or unstable deformation results, including unexpected global changes outside the region of interest. To address this fundamental limitation, we propose a sparse blending method that automatically selects a smaller number of deformation modes to compactly describe the desired deformation. This along with a suitably chosen deformation basis including spatially localized deformation modes leads to significant advantages, including more meaningful, reliable, and efficient deformations because fewer and localized deformation modes are applied. To cope with large rotations, we develop a simple but effective representation based on polar decomposition of deformation gradients, which resolves the ambiguity of large global rotations using an as-consistent-as-possible global optimization. This simple representation has a closed form solution for derivatives, making it efficient for our sparse localized representation and thus ensuring interactive performance. Experimental results show that our method outperforms state-of-the-art data-driven mesh deformation methods, for both quality of results and efficiency.
» Show BibTeX
@article{gao2019sparse,
title={Sparse data driven mesh deformation},
author={Gao, Lin and Lai, Yu-Kun and Yang, Jie and Ling-Xiao, Zhang and Xia, Shihong and Kobbelt, Leif},
journal={IEEE transactions on visualization and computer graphics},
year={2019},
publisher={IEEE}
}
Active Exploration of Large 3D Model Repositories
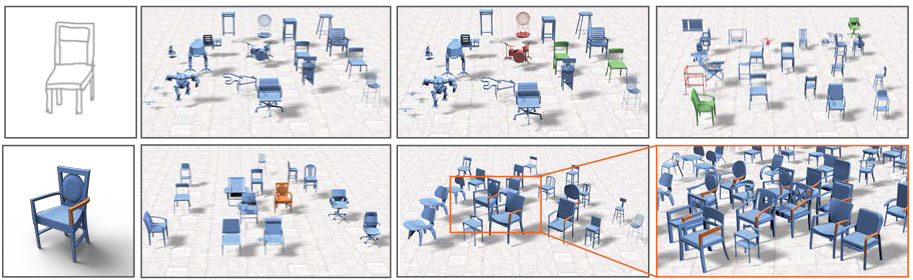
With broader availability of large-scale 3D model repositories, the need for efficient and effective exploration becomes more and more urgent. Existing model retrieval techniques do not scale well with the size of the database since often a large number of very similar objects are returned for a query, and the possibilities to refine the search are quite limited. We propose an interactive approach where the user feeds an active learning procedure by labeling either entire models or parts of them as “like” or “dislike” such that the system can automatically update an active set of recommended models. To provide an intuitive user interface, candidate models are presented based on their estimated relevance for the current query. From the methodological point of view, our main contribution is to exploit not only the similarity between a query and the database models but also the similarities among the database models themselves. We achieve this by an offline pre-processing stage, where global and local shape descriptors are computed for each model and a sparse distance metric is derived that can be evaluated efficiently even for very large databases. We demonstrate the effectiveness of our method by interactively exploring a repository containing over 100K models.
» Show BibTeX
@ARTICLE{6951464,
author={L. {Gao} and Y. {Cao} and Y. {Lai} and H. {Huang} and L. {Kobbelt} and S. {Hu}},
journal={IEEE Transactions on Visualization and Computer Graphics},
title={Active Exploration of Large 3D Model Repositories},
year={2015},
volume={21},
number={12},
pages={1390-1402},}