Profile
![]() |
Publications
Neural Implicit Shape Editing Using Boundary Sensitivity
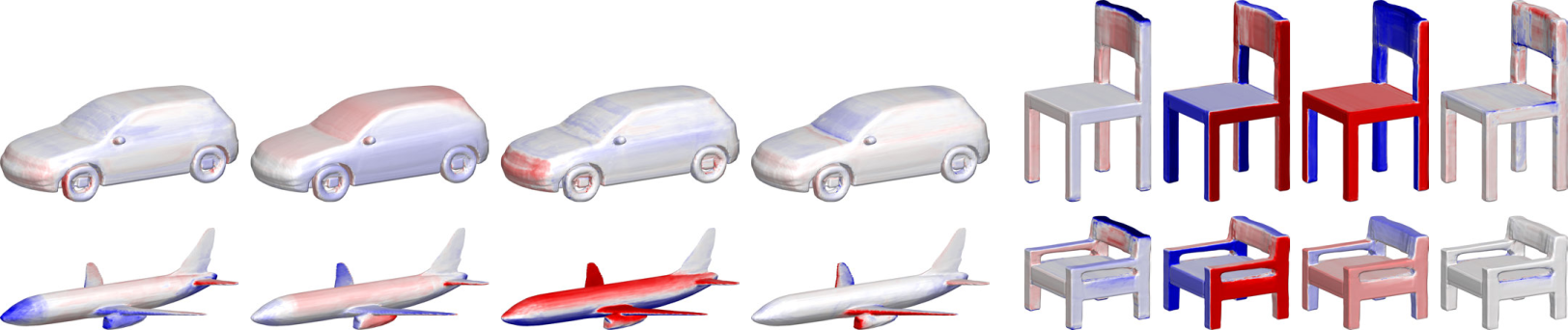
Neural fields are receiving increased attention as a geometric representation due to their ability to compactly store detailed and smooth shapes and easily undergo topological changes. Compared to classic geometry representations, however, neural representations do not allow the user to exert intuitive control over the shape. Motivated by this, we leverage boundary sensitivity to express how perturbations in parameters move the shape boundary. This allows to interpret the effect of each learnable parameter and study achievable deformations. With this, we perform geometric editing: finding a parameter update that best approximates a globally prescribed deformation. Prescribing the deformation only locally allows the rest of the shape to change according to some prior, such as semantics or deformation rigidity. Our method is agnostic to the model its training and updates the NN in-place. Furthermore, we show how boundary sensitivity helps to optimize and constrain objectives (such as surface area and volume), which are difficult to compute without first converting to another representation, such as a mesh.
@misc{berzins2023neural,
title={Neural Implicit Shape Editing using Boundary Sensitivity},
author={Arturs Berzins and Moritz Ibing and Leif Kobbelt},
year={2023},
eprint={2304.12951},
archivePrefix={arXiv},
primaryClass={cs.CV}
}
Octree Transformer: Autoregressive 3D Shape Generation on Hierarchically Structured Sequences
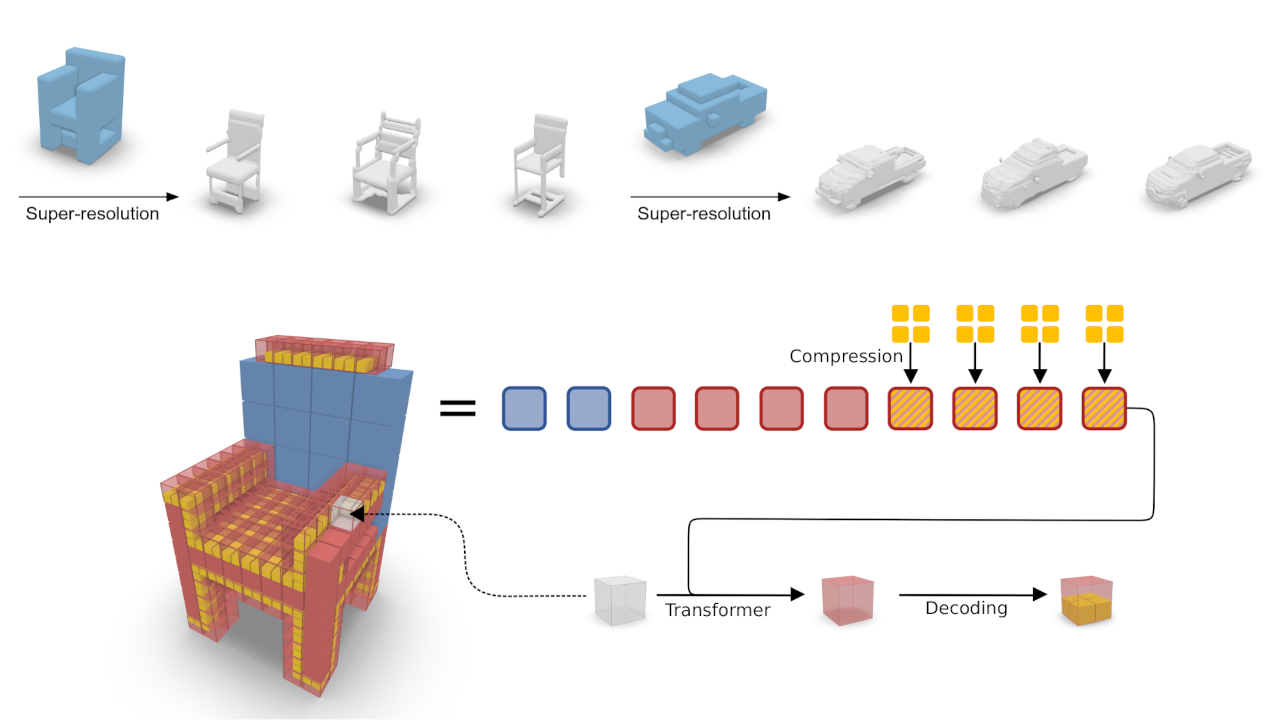
Autoregressive models have proven to be very powerful in NLP text generation tasks and lately have gained pop ularity for image generation as well. However, they have seen limited use for the synthesis of 3D shapes so far. This is mainly due to the lack of a straightforward way to linearize 3D data as well as to scaling problems with the length of the resulting sequences when describing complex shapes. In this work we address both of these problems. We use octrees as a compact hierarchical shape representation that can be sequentialized by traversal ordering. Moreover, we introduce an adaptive compression scheme, that significantly reduces sequence lengths and thus enables their effective generation with a transformer, while still allowing fully autoregressive sampling and parallel training. We demonstrate the performance of our model by performing superresolution and comparing against the state-of-the-art in shape generation.
@inproceedings{ibing_octree,
author = {Moritz Ibing and
Gregor Kobsik and
Leif Kobbelt},
title = {Octree Transformer: Autoregressive 3D Shape Generation on Hierarchically Structured Sequences},
booktitle = {{IEEE/CVF} Conference on Computer Vision and Pattern Recognition Workshops,
{CVPR} Workshops 2023},
publisher = {{IEEE}},
year = {2023},
}
Localized Latent Updates for Fine-Tuning Vision-Language Models
Although massive pre-trained vision-language models like CLIP show impressive generalization capabilities for many tasks, still it often remains necessary to fine-tune them for improved performance on specific datasets. When doing so, it is desirable that updating the model is fast and that the model does not lose its capabilities on data outside of the dataset, as is often the case with classical fine-tuning approaches. In this work we suggest a lightweight adapter that only updates the models predictions close to seen datapoints. We demonstrate the effectiveness and speed of this relatively simple approach in the context of few-shot learning, where our results both on classes seen and unseen during training are comparable with or improve on the state of the art.
@inproceedings{ibing_localized,
author = {Moritz Ibing and
Isaak Lim and
Leif Kobbelt},
title = {Localized Latent Updates for Fine-Tuning Vision-Language Models},
booktitle = {{IEEE/CVF} Conference on Computer Vision and Pattern Recognition Workshops,
{CVPR} Workshops 2023},
publisher = {{IEEE}},
year = {2023},
}
3D Shape Generation with Grid-based Implicit Functions
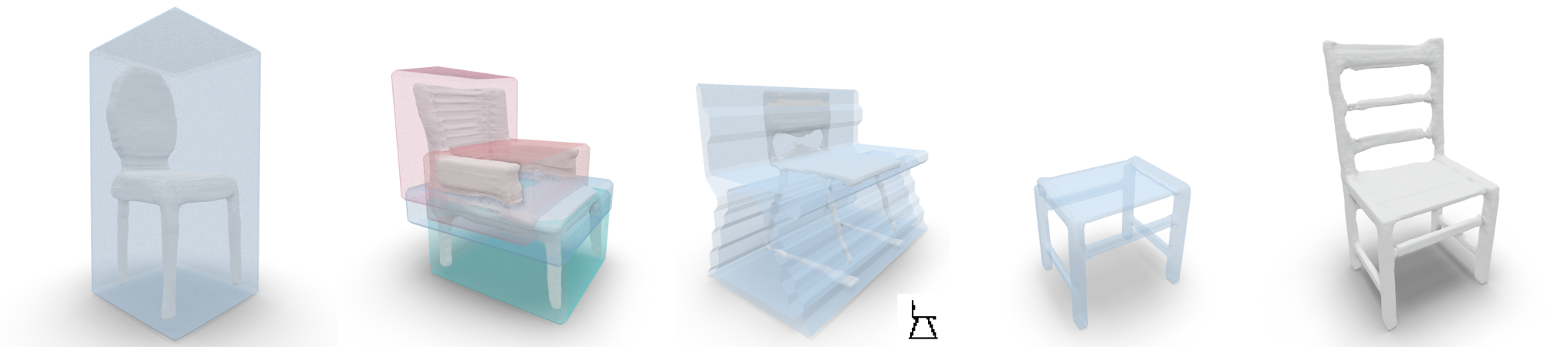
Previous approaches to generate shapes in a 3D setting train a GAN on the latent space of an autoencoder (AE). Even though this produces convincing results, it has two major shortcomings. As the GAN is limited to reproduce the dataset the AE was trained on, we cannot reuse a trained AE for novel data. Furthermore, it is difficult to add spatial supervision into the generation process, as the AE only gives us a global representation. To remedy these issues, we propose to train the GAN on grids (i.e. each cell covers a part of a shape). In this representation each cell is equipped with a latent vector provided by an AE. This localized representation enables more expressiveness (since the cell-based latent vectors can be combined in novel ways) as well as spatial control of the generation process (e.g. via bounding boxes). Our method outperforms the current state of the art on all established evaluation measures, proposed for quantitatively evaluating the generative capabilities of GANs. We show limitations of these measures and propose the adaptation of a robust criterion from statistical analysis as an alternative.
@inproceedings {ibing20213Dshape,
title = {3D Shape Generation with Grid-based Implicit Functions},
author = {Ibing, Moritz and Lim, Isaak and Kobbelt, Leif},
booktitle = {IEEE Computer Vision and Pattern Recognition (CVPR)},
pages = {},
year = {2021}
}
Intuitive Shape Editing in Latent Space
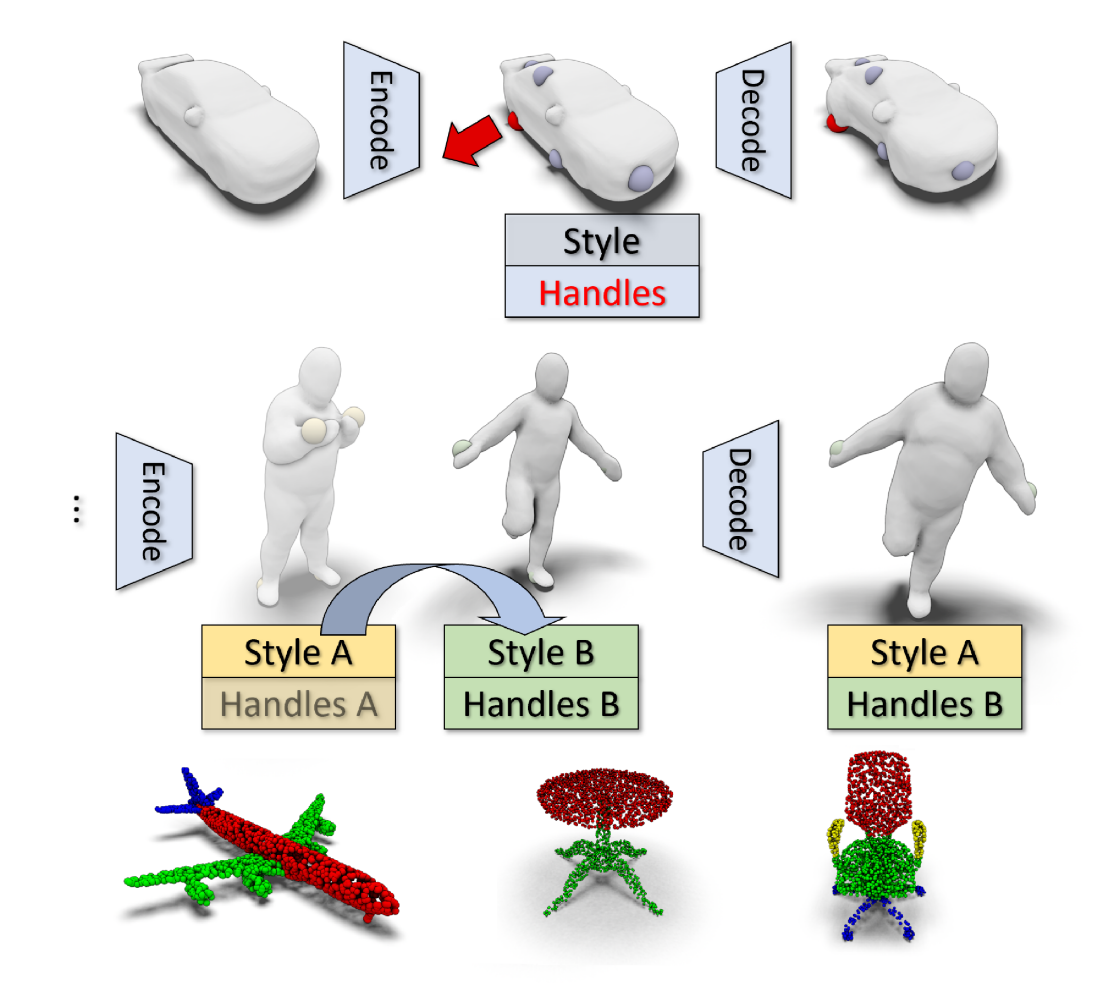
The use of autoencoders for shape editing or generation through latent space manipulation suffers from unpredictable changes in the output shape. Our autoencoder-based method enables intuitive shape editing in latent space by disentangling latent sub-spaces into style variables and control points on the surface that can be manipulated independently. The key idea is adding a Lipschitz-type constraint to the loss function, i.e. bounding the change of the output shape proportionally to the change in latent space, leading to interpretable latent space representations. The control points on the surface that are part of the latent code of an object can then be freely moved, allowing for intuitive shape editing directly in latent space. We evaluate our method by comparing to state-of-the-art data-driven shape editing methods. We further demonstrate the expressiveness of our learned latent space by leveraging it for unsupervised part segmentation.
A Convolutional Decoder for Point Clouds using Adaptive Instance Normalization
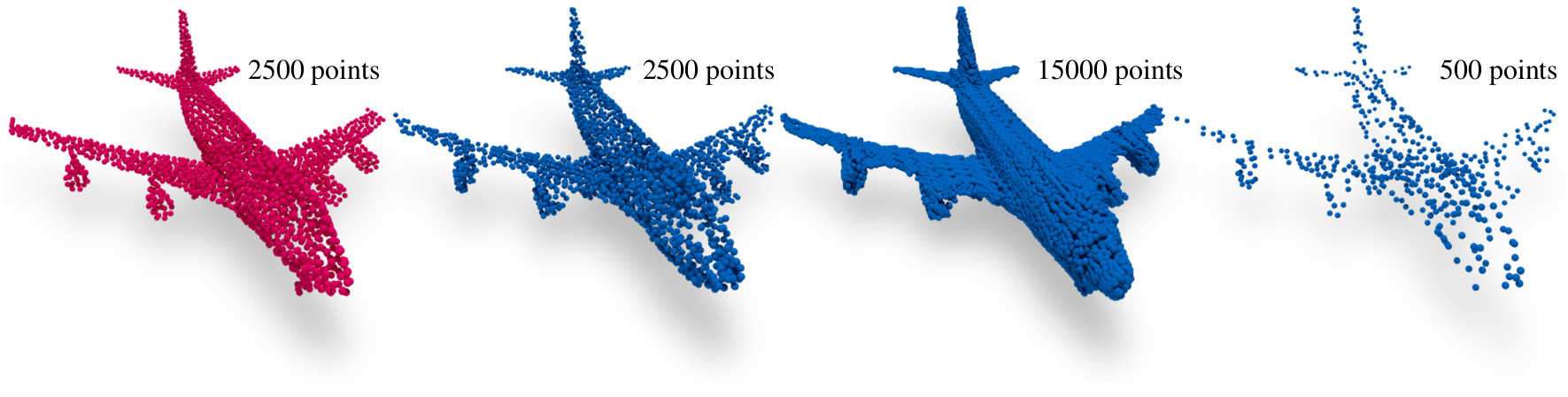
Automatic synthesis of high quality 3D shapes is an ongoing and challenging area of research. While several data-driven methods have been proposed that make use of neural networks to generate 3D shapes, none of them reach the level of quality that deep learning synthesis approaches for images provide. In this work we present a method for a convolutional point cloud decoder/generator that makes use of recent advances in the domain of image synthesis. Namely, we use Adaptive Instance Normalization and offer an intuition on why it can improve training. Furthermore, we propose extensions to the minimization of the commonly used Chamfer distance for auto-encoding point clouds. In addition, we show that careful sampling is important both for the input geometry and in our point cloud generation process to improve results. The results are evaluated in an auto-encoding setup to offer both qualitative and quantitative analysis. The proposed decoder is validated by an extensive ablation study and is able to outperform current state of the art results in a number of experiments. We show the applicability of our method in the fields of point cloud upsampling, single view reconstruction, and shape synthesis.
@article{Lim:2019:ConvolutionalDecoder,
author = "Lim, Isaak and Ibing, Moritz and Kobbelt, Leif",
title = "A Convolutional Decoder for Point Clouds using Adaptive Instance Normalization",
journal = "Computer Graphics Forum",
volume = 38,
number = 5,
year = 2019
}
Scale-Invariant Directional Alignment of Surface Parametrizations
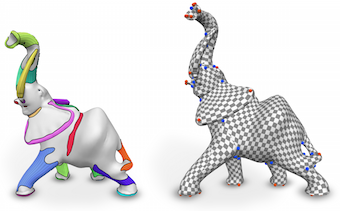
Various applications of global surface parametrization benefit from the alignment of parametrization isolines with principal curvature directions. This is particularly true for recent parametrization-based meshing approaches, where this directly translates into a shape-aware edge flow, better approximation quality, and reduced meshing artifacts. Existing methods to influence a parametrization based on principal curvature directions suffer from scale-dependence, which implies the necessity of parameter variation, or try to capture complex directional shape features using simple 1D curves. Especially for non-sharp features, such as chamfers, fillets, blends, and even more for organic variants thereof, these abstractions can be unfit. We present a novel approach which respects and exploits the 2D nature of such directional feature regions, detects them based on coherence and homogeneity properties, and controls the parametrization process accordingly. This approach enables us to provide an intuitive, scale-invariant control parameter to the user. It also allows us to consider non-local aspects like the topology of a feature, enabling further improvements. We demonstrate that, compared to previous approaches, global parametrizations of higher quality can be generated without user intervention.
@article{Campen:2016:ScaleInvariant,
author = "Campen, Marcel and Ibing, Moritz and Ebke, Hans-Christian and Zorin, Denis and Kobbelt, Leif",
title = "Scale-Invariant Directional Alignment of Surface Parametrizations",
journal = "Computer Graphics Forum",
volume = 35,
number = 5,
year = 2016
}